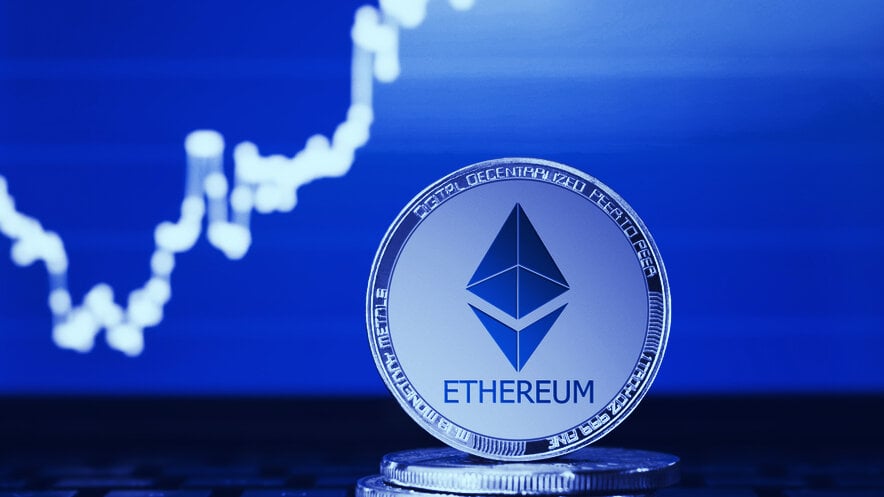
The price of Ethereum has increased by 11% in the past 24 hours to highs of $2,640. The rise caps off a 24% increase in the past week.
Ethereum has brought several coins that use its smart contracts along for the ride. Most of the top decentralized finance (DeFi) tokens have also increased in price in the past day.
DeFi growth
Uniswap (UNI), which has a market cap of $15.6 billion and is the largest DeFi token by this measure, has risen by 12% in the past day. At a price of $27, the coin, which powers the governance protocol of the eponymous decentralized exchange, has increased by nearly 28% during the past week.
Chainlink (LINK)—UNI’s closest competitor with a market cap of $13.4 billion—has increased by 15% today and by a staggering 32% during the past week. Currently, LINK, which powers a decentralized oracle network, is worth $31.
Other DeFi tokens have also grown in the past week. Aave (AAVE) and Maker (MKR) have increased by just over 10% in the past day and by 6% and 1% over the past seven days, respectively.
Wider market jubilance
The crypto market’s largest DeFi tokens might be going up, but they’re not the only cryptocurrencies that are on the rise.
Despite renewed scrutiny on its carbon footprint, Bitcoin is also increasing in value. The flagship cryptocurrency—currently priced at $36,900—has increased by almost 6% during the last week and 4% during the last 24 hours.
XRP, the sixth-largest cryptocurrency by market cap, has surged in price in the past few days. Now valued at $1.02, XRP has grown by 15% today and 27% during the last week.
XRP’s rise follows Ripple’s recent legal victory against the US Securities and Exchange Commission, which in December 2020 filed a lawsuit alleging that Ripple raised $1.3 billion by selling XRP in unregistered securities sales.
On Sunday, Ripple, the payments company whose co-founders created XRP, stopped the regulator from accessing records of advice that Ripple has sought or received about the legal status of XRP.